On Sentiment Analysis and Transformative Methods in Digital Humanities
By Debbie Brubaker, Mellon Graduate Fellow, Religion/Theological Studies
When digital technologies are integrated into humanistic research projects, the search for alignment between research tools and objectives often challenges commonly-used approaches. This has been my experience during data collection, corpus creation, and through the selection of technical tools as a Mellon Graduate Fellow in DH. Most recently, my exposure to sentiment analysis has demonstrated that both humanistic methods and digital tools may require significant modifications when DH research methods are employed.
Sentiment analysis is a way of using computers to evaluate the emotion or connotation of words within texts.[1] Digital tools read a text and assign a sentiment value to phrases and words. These values categorize words on a scale of negative to positive sentiment. Values are determined with the use of “sentiment lexicons.” The lexicons operate like a dictionary or code book, with a catalog of words with an assigned sentiment value. Sentiment analysis tools then scan texts and assign sentiment value to words and phrases according to the sentiment lexicon employed by the tool.
Yet, sentiment is context-specific. The sentiment, the emotion, the feel of a word depends on context. Sentiment relies not just on a word’s proximity to other words, but also its usage domain, its social context. This includes the conventions that guide the mode and medium of its delivery. Think how the sentiment of “crazy” might differ from its use in a tweet, to a line in a novel, or how the sentiment of “crazy” might change over the span of decades. These are nuances that humanistic inquiry knows well, but what about computer programs?
The variability of sentiment has pushed researchers to develop new domain-specific sentiment lexicons. These are sentiment lexicons that assign value to words based on the social or time-bound use of words. For example, Hamilton et.al. have released historical lexicons for 15 decades of English and community-specific lexicons for users communicating on Reddit. These lexicons are available online and can be used to perform sentiment analysis.
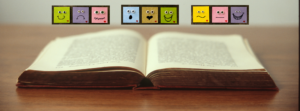
But what happens when the existing sentiment lexicons don’t fit your project? Does it matter if we conduct sentiment analysis on texts with lexicons that don’t have a domain match? And what happens if our dataset originates from multiple domains?
My own research of Christian communities requires paying attention to these questions. In my work I analyze the transcripts of digital artifacts—audio, visual, and textual content—produced and posted online by U.S. Christian churches. These transcriptions represent previously recorded, in-person worship services, sermons, and presentations. The words in the transcripts capture a variety of content, from lyrics of songs, to the prayers of congregants, and scripture recited in the homily.
The digital artifacts I examine record a wide variety of activities, but this isn’t the only source of heterogeneity in my corpus. The communities I study have a wide range of technical proficiency. These digital artifacts are structured according to the functions they serve for the community. Additionally, there are various platforms where media is posted, sometimes determining length or form. And further, the web-scraping and transcription techniques used to retrieve and transcribe these digital artifacts recontextualize these complex digital objects and events into texts that are readable for computer analysis. This raises one question: do the textual objects I’ve created have the same “usage domain” as the digital artifacts from which they originate?
I’m still working through this question. But let’s say for the moment that these texts originate from a heterogeneous, though identifiable digital Christian domain. Now, let’s go back to sentiment lexicons.
The texts I’m reading aren’t a collection of tweets, or Reddit posts, but they are social media. These texts aren’t novels or newspapers, but sometimes they are edited, scripted, and quite formal. Though “born digital,” they relay patterns and norms that scholars in Christian ritual studies have spent much time identifying and dissecting.
So, does it make sense to use sentiment lexicons based on contemporary literature or social media to perform sentiment analysis of these digital artifacts? Do I apply a tool meant for another context and hope that it creates new entry points for evaluating my artifacts? Do I run analysis using multiple existing sentiment lexicons? Or, do I stall analysis of my corpus to develop a domain-specific sentiment lexicon? And if I develop a domain-specific sentiment lexicon, how should existing humanistic approaches to Christian thought and practice inform this lexicon?
For me, how these questions are addressed has much to do with the relationship between quantitative data science and humanistic study of religious phenomena. Or said differently, it concerns the relationship between “digital tech” and “humanities” in what we call “digital humanities.”
In a talk last year called “The Dangerous Art of Text Mining” (also the title of a forthcoming book), Jo Guldi offers an intriguing approach to such questions. Likening an archive (she’s an historian) to a sculpture, she demonstrates how several strategic approaches are needed for the comprehension of multidimensional research objects. She advocates an “ambling, iterative course of exploration” that promotes “hybrid knowledge” where humanistic interpretation and quantitative analysis operate dialogically. For Guldi, this means methods of historical interpretation inform the selection and use of algorithms. And yet, the algorithms also direct interpretive routes of inquiry and reflection. Always, the process repeats with a difference. Variance and limitations are noted by using different tools, so methodological plurality is valuable; different approaches and techniques are needed. What she advocates as methods of “Critical Search” and “hybrid knowledge” involves multi-dimensional, iterative interpretation and analysis to make sense of research objects.
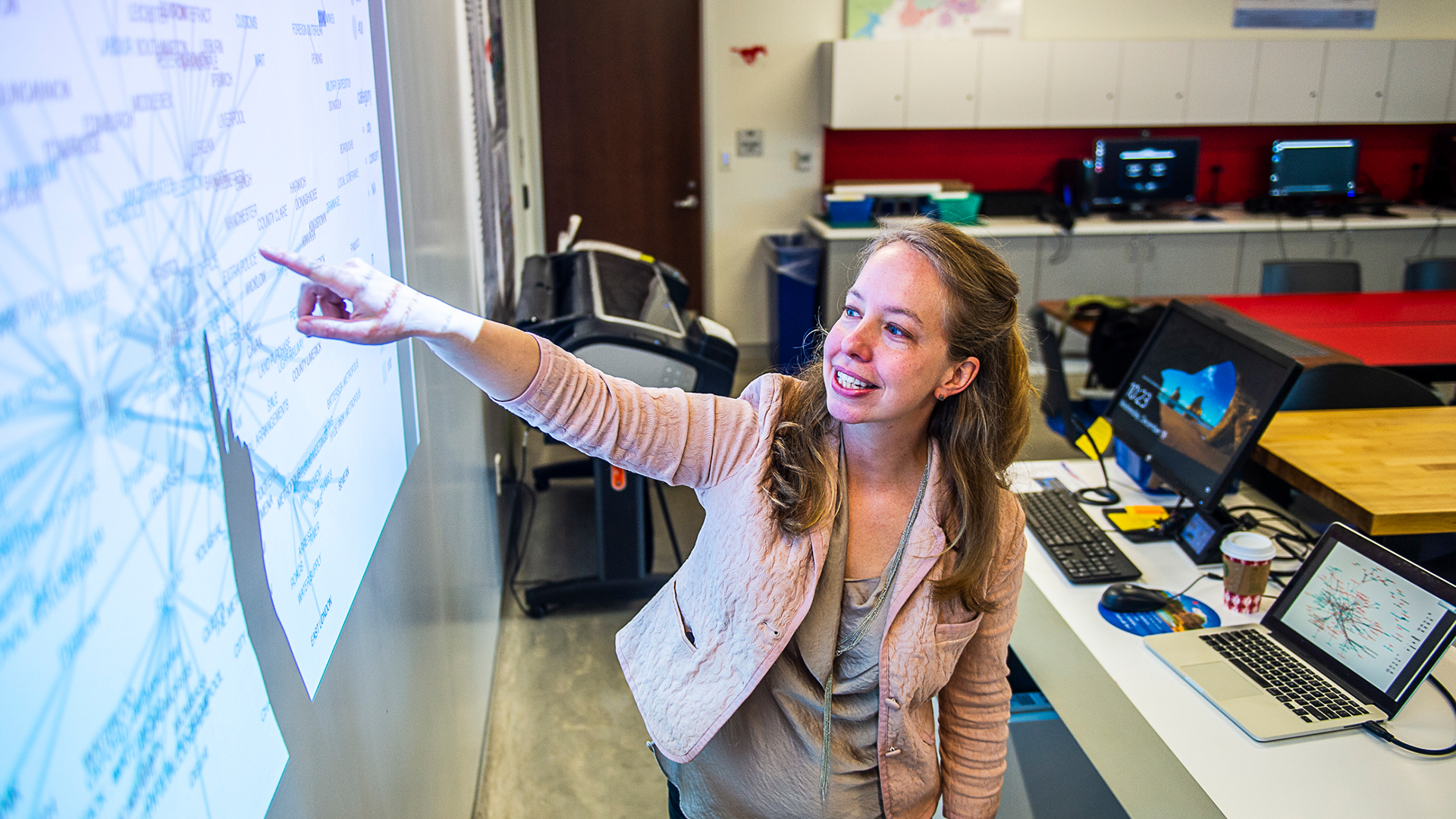
There’s a lot to digest in Guldi’s work, but for me, this is one takeaway: we need to critically interrogate how our disciplinary training transforms and is transformed by our use of digital technologies. Attention to this exchange reveals how modes of critical humanistic inquiry challenge the design and structure of quantitative tools.[2] For example, when a historian can identify gaps or occlusions in an archive that an algorithm cannot; or when phenomenological accounts of religious experience demand and direct domain-specific sentiment lexicons.
In my own analysis of digital media produced by U.S. Christian churches, experimentation with digital tools like sentiment analysis opened new routes of research, and indeed has even challenged the stability of my research object entirely. This has necessitated critical reflection about the revelatory interaction between my religious studies approach and the algorithmic structures of my digital tools. This type of critical reflection on methodological limitations, possibilities, and interactions can be transformative for our work as digital humanists.
—-
[1] There are also forms of sentiment analysis that examine audio recordings and visual objects.
[2] This type of reflection also helps to identify moments when uncritical use of digital technologies reproduces bias or violence, as my colleague Katerina Trout’s most recent blog post addresses.