Hannah Weeks dissertation defense – October 24
PhD candidate Hannah L. Weeks will defend her dissertation on Tuesday, October 24, at 10 a.m. Central Time, at 2525 West End Avenue, in the 10th floor conference room (suite 1020, room 10105). Her advisor is Matthew Shotwell. All are invited and encouraged to attend.
Overcoming Challenges with Real World Data and Clinical Restrictions in Pharmacokinetic Analyses
Real world observational pharmacokinetic (PK) data—for example, from electronic health records or clinical measurements collected during the course of routine care—require additional analysis considerations relative to traditional PK trials. Data from real patients are more likely to be sparse, imbalanced, or error-prone, and the practical limitations must be considered to implement methods in a clinical setting. In this dissertation, we developed and investigated methodologies to improve studies conducted using real world PK data. First, we developed a natural language processing algorithm, medExtractR, which targets the extraction of dose information from free-text clinical notes for a specific medication of interest. Contrasted with existing general-purpose medication extraction algorithms, medExtractR was able to achieve higher entity-level extraction, and maintained high performance on an external validation set with tuning and customization. Next, we examined an approximate Bayesian model for individualized PK estimation via a simulation study with opportunistic concentration measurements. The intended use case of therapeutic drug monitoring necessitated approximation methods that were both accurate and could be implemented in a computationally efficient manner. We evaluated model performance, including accuracy of uncertainty estimation, and implemented the methods into an easy-to-use web interface. Finally, we addressed the issue of bias in estimation as a result of time recording errors in PK data by evaluating various modifications to study design and data collection. We considered pragmatic strategies minimally invasive to a clinical workflow, such that they could be implemented in an opportunistic data setting. Mitigation strategies included delaying timing of blood draws to non-infusion periods, selecting future draw times based on minimizing bias from simulated errors, and identifying patients whose existing measurements were most sensitive to recording errors. For an intravenously infused drug, we found that these strategies generally reduced bias in estimation of a pharmacodynamic endpoint for dosing schedules with short, rapid infusions. These strategies were less helpful in reducing bias for schedules with longer, slower infusions, which were less sensitive to the presence of recording errors in general.
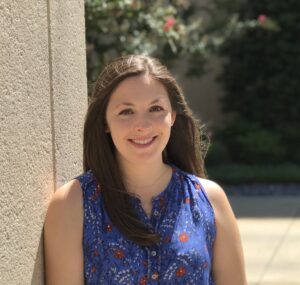